
We support all annotation types
Our high performing professionals use AI-enabled tools to create bounding boxes and segmentation that accelerate training data requirements with highest quality, accuracy and scalability. Our combination of humans and machine learning is built to solve a wide range of use cases. Our goal is to power innovation at all places.

Bounding Boxes
Annotating images or videos with bounding boxes around objects of choice. Widely used to train autonomous driving prediction models for lanes, pedestrians, cyclists, vehicles, etc. Computer vision models for spatial cognition from 2D images or videos and Lane detection for AV’s.

Polygons
Drawing polygons by identifying the exact shape of the object in aerial and satellite imagery. Usually used to mark the shape of an irregular object which cannot be accurately captured by bounding boxes with precision. Polygons are useful for creating training data sets for AI and machine learning in multiple industries.
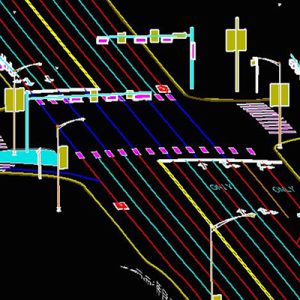
Polylines
Feature used for labeling GIS data like roads, lanes, highways, airstrips, rail road’s, coastal line etc. The most common application of lane annotation is for autonomous vehicle. By annotating road lanes and sidewalks, the autonomous vehicle can be trained to understand boundaries.
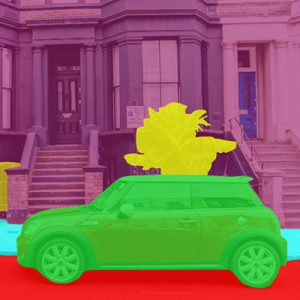
Semantic Segmentation
Semantic Segmentation is an aspect of image processing and computer vision process used to locate objects and boundaries defined in real world as two dimensional function. Semantic segmentation is the process of linking each pixel in an image to a class label. These labels could include a person, car, flower, piece of furniture, etc on roads, in retail shops.
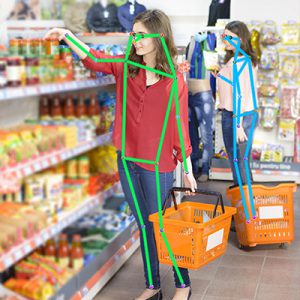
Key Point Annotation
Key point annotation are used to label facial/skeletal features, automotive parts etc. in an image. We label images using points to determine the shape of it. We provide Key Point annotation in human faces by marking facial features, joint positions, using single points for detection of emotions and facial recognition such as for retail, supermarkets and grocery stores.
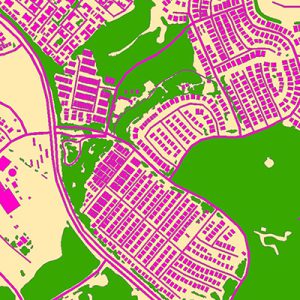
Geo-Annotation
We create geographic models using remote sensing technology, such as satellite, aerial, and drone imagery, to produce and curate training data for machine learning and computer vision models. Geo-annotation is useful for aerial view and drone imagery annotation, bounding box, semantic segmentation, etc.