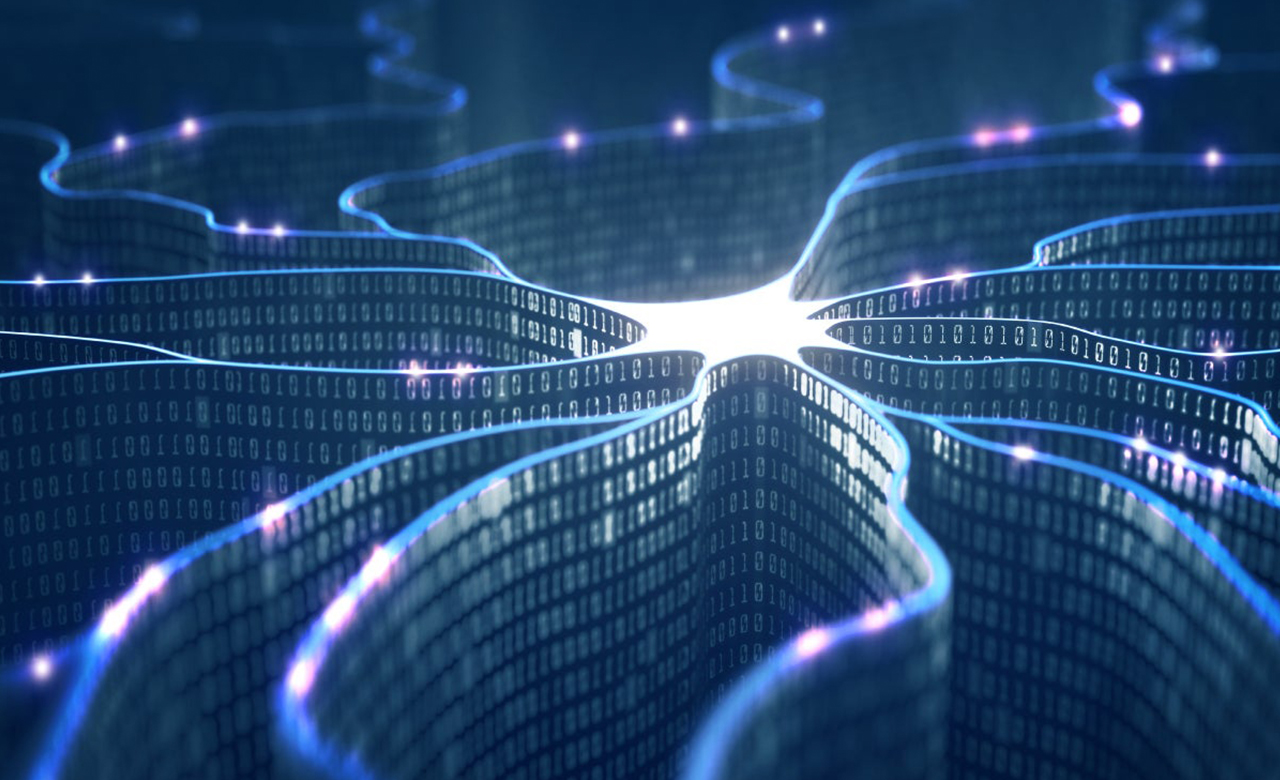
Natural catastrophes, from hurricanes and earthquakes to floods and wildfires, have the potential to wreak havoc on communities and economies. As the frequency and intensity of these events continue to rise, accurate modeling becomes increasingly crucial for effective risk assessment and mitigation strategies. However, the reliability of natural catastrophe models hinges on the quality of the underlying data. This is where data cleansing plays a pivotal role, ensuring that the input data is accurate, consistent, and reliable. Inaccurate or outdated information can lead to flawed outcomes, expensive reinsurance placement, or non-placement of optimum capacity.

One of the most important needs for insurers is to have multi-model access so that they are able to get more data points to make crucial pricing and reserving decisions. Different natural catastrophe models rely on multiple datasets from various sources. RMS’s RiskLink uses EDM, VERISK’s models use CEDE data format and the Oasis-based models use OED data formats. However, in order to exploit the multi-modeling capability, it is imperative to have the ability to transform the available data seamlessly into different model-ready formats.
RMSI took a pivotal step in ensuring the integrity of the conversion of data across different models by banking on the transformative power of artificial intelligence (AI). Our data cleansing is backed by AI which is trained on vast databases of insurance data formats available with RMSI and in the open-source environment.
RMSI’s data cleansing process leverages AI to cut down on manual tasks and provide scale to ensure quick turnaround time at optimum cost. Our process includes the following:
Analyzing the data
The journey begins with a sophisticated analysis of the available data formats empowered by generative AI. By employing machine learning algorithms, this stage enhances the accuracy of data quality assessments. Generative AI not only identifies patterns but also discerns anomalies, automating the detection of errors and inconsistencies.
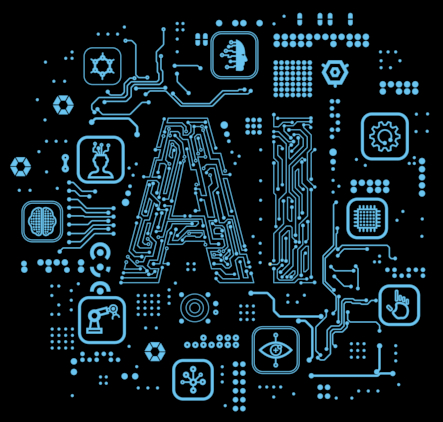
Gap identification across data sets
By utilizing advanced algorithms, the system identifies missing or incomplete data points and generates a comprehensive action report. Gaps such as missing modifier details, incorrect or incomplete coding, and incorrect address formatting are flagged. Our approach ensures a proactive response to data gaps, mitigating potential issues before they impact the modeling process.
Geocode
The geocoding phase is revolutionized with the implementation of auto-geocoding. Prebuilt algorithms automate the process of assigning geographic coordinates to risk locations, streamlining spatial refinement and ensuring accurate representation of data across diverse datasets.
Modifier updation
This stage ensures the auto-coding of primary modifiers. Leveraging machine learning models, this phase ensures the efficient and accurate assignment of modifiers to relevant data elements, reducing manual efforts and enhancing the overall consistency of the dataset.

Apply reinsurance structures
Rule-based transformation, facilitated by AI, is employed to apply reinsurance structures. Machine learning algorithms process complex rule sets, ensuring a seamless integration of reinsurance structures into the data. The challenge of mapping the fields, data types, and structures from one format taking into account the inconsistencies in naming conventions or data hierarchies is solved through a proper mapping of both the data sets powered by our AI algorithms. This AI-driven approach enhances the accuracy and efficiency of the transformation process.
Transform
The final transformation phase is powered through facilitation by our machine learning models which ensures that the output aligns seamlessly with the target format requirements. This AI-driven transformation guarantees accuracy and consistency in the final dataset.

Data cleansing is the cornerstone of reliable and accurate natural catastrophe modeling. By addressing issues related to accuracy, consistency, and quality, practitioners can utilize robust models that enhance our understanding of risk and facilitate effective reinsurance strategies. As these catastrophic events continue to rise putting stress on the bottom line of reinsurers, the role of data cleansing in catastrophe modeling becomes increasingly critical for informed decision-making and sustainable risk management.